What It Does and the Key Benefits It Offers
We create custom machine-learning models to optimize industrial processes in real time.
Reduce energy consumption and CO2 emissions
Improve product quality
Minimize raw material costs
Integrate easily into existing data infrastructure
Case Studies
EAF – Energy Consumption Reduction
Metalfer, Serbia
Predicting energy consumption using ~40 process parameters → led to energy savings.
Finished project
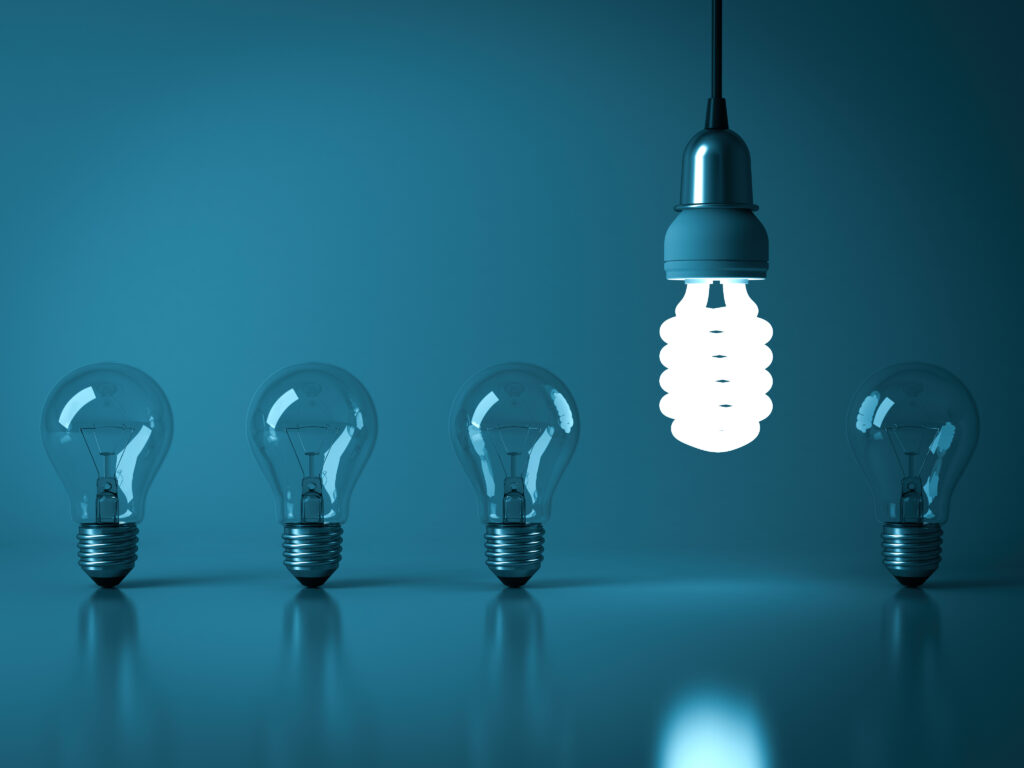
EAF – Raw Materials Savings
Metalfer, Serbia
ML method to reduce alloying elements (Mn, Si) by changing process practice.
Finished project
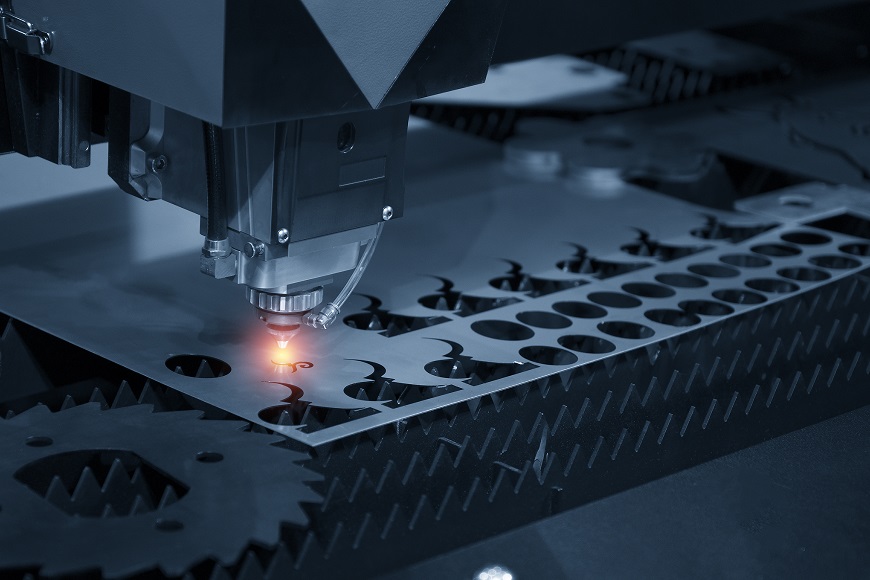
EAF – Mechanical Properties
Metalfer, Serbia
Impact of tramp elements on tensile strength to tune process parameters.
Ongoing
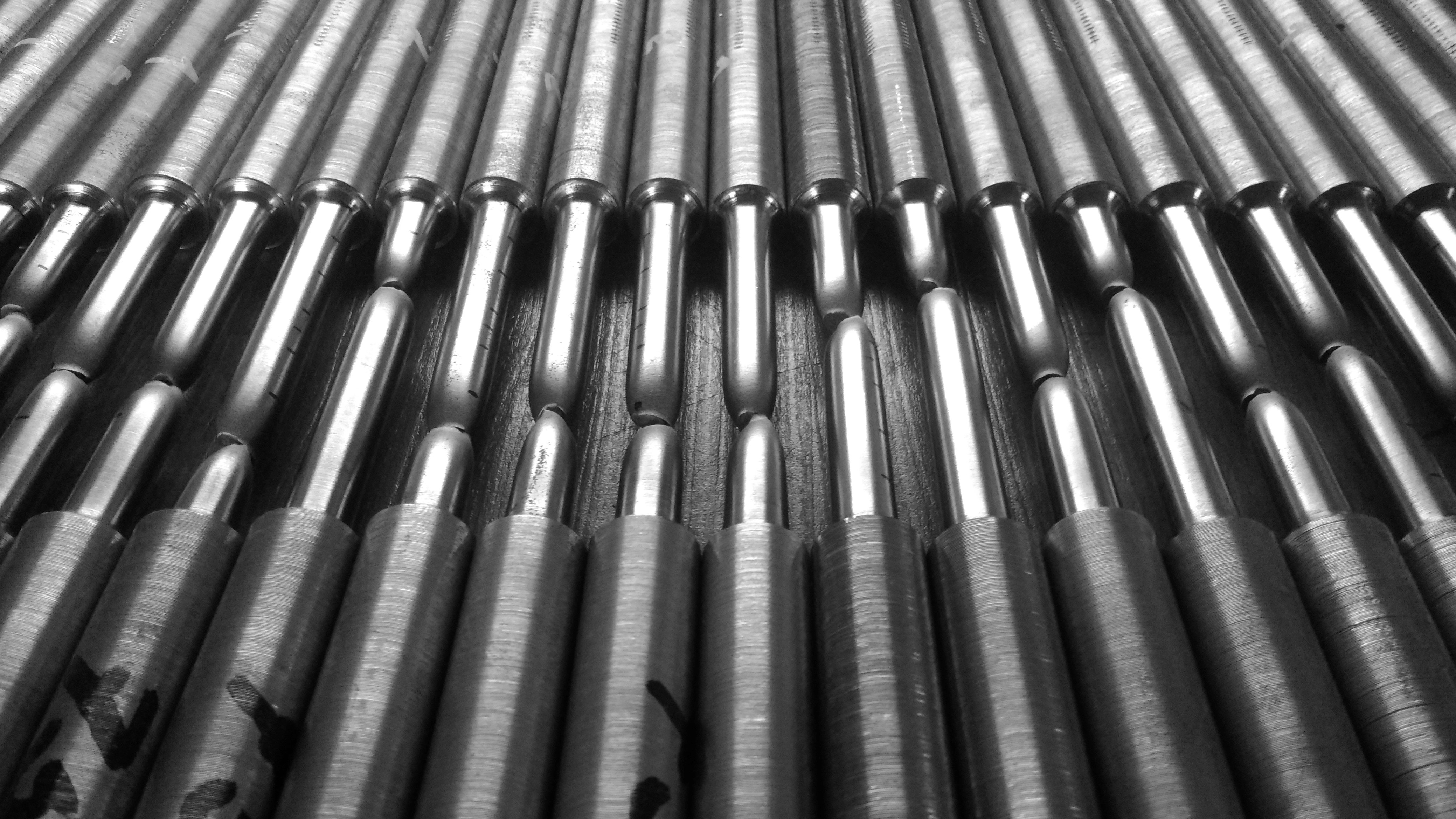
EAF – Lime and Carbon Injection
Metalfer, Serbia
Optimizing slag foaming with an ML model and new injection equipment.
Ongoing
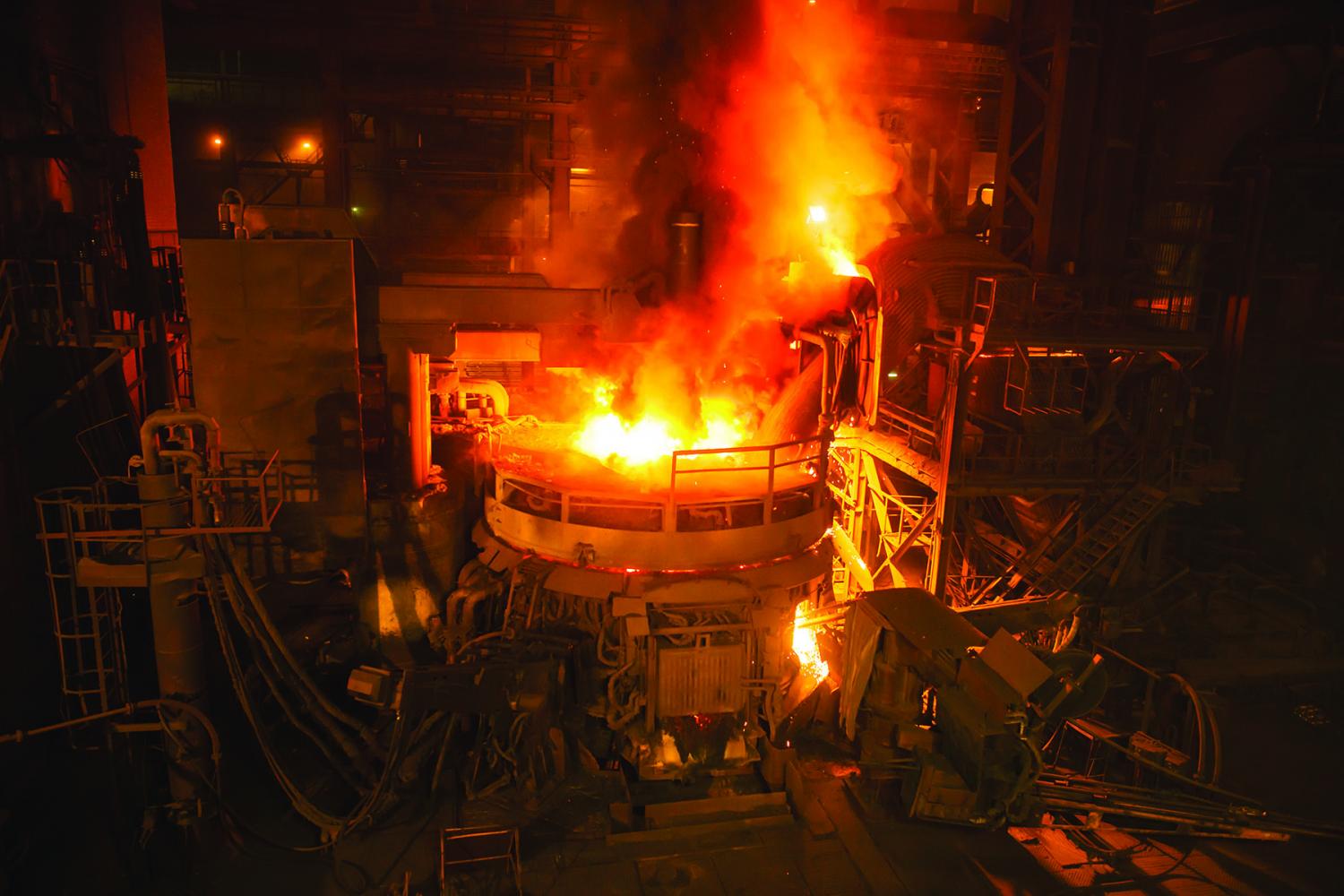
Energy Revision – HBIS, Serbia
(by University of Belgrade, Faculty of Technology and Metallurgy)
Monitoring energy consumption in multiple processing units to find leaks and savings.
Finished
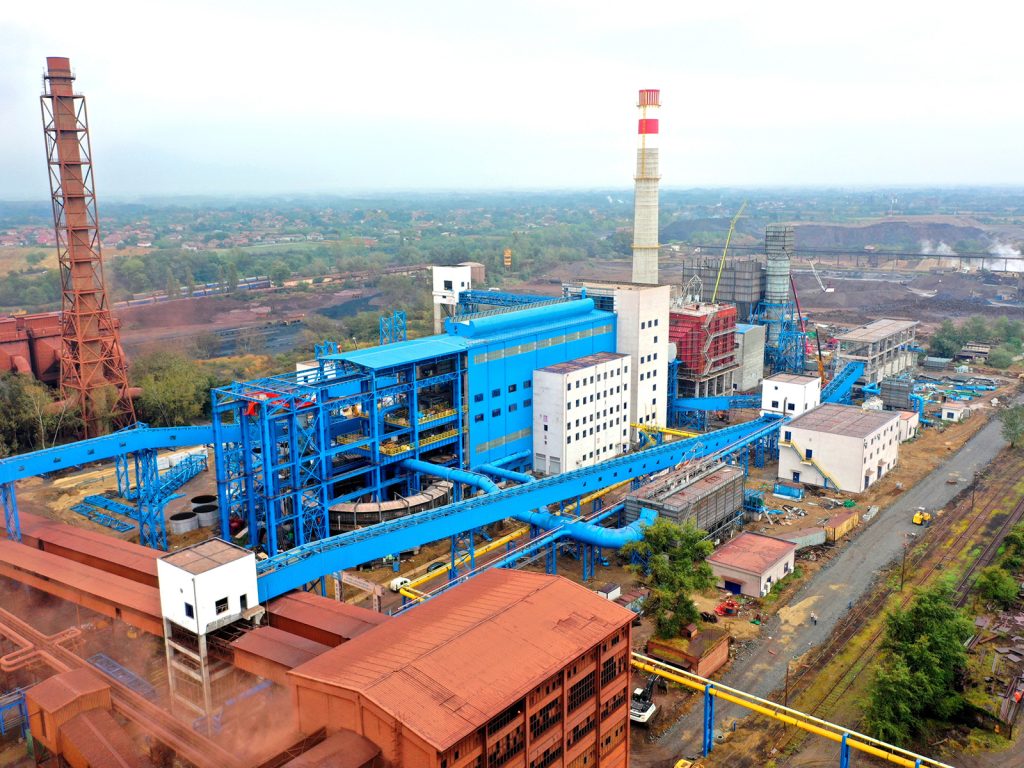
Material Savings via Microalloying
HBIS, Serbia
Predicting mechanical properties by replacing large amounts of Mn with microelements.
Ongoing
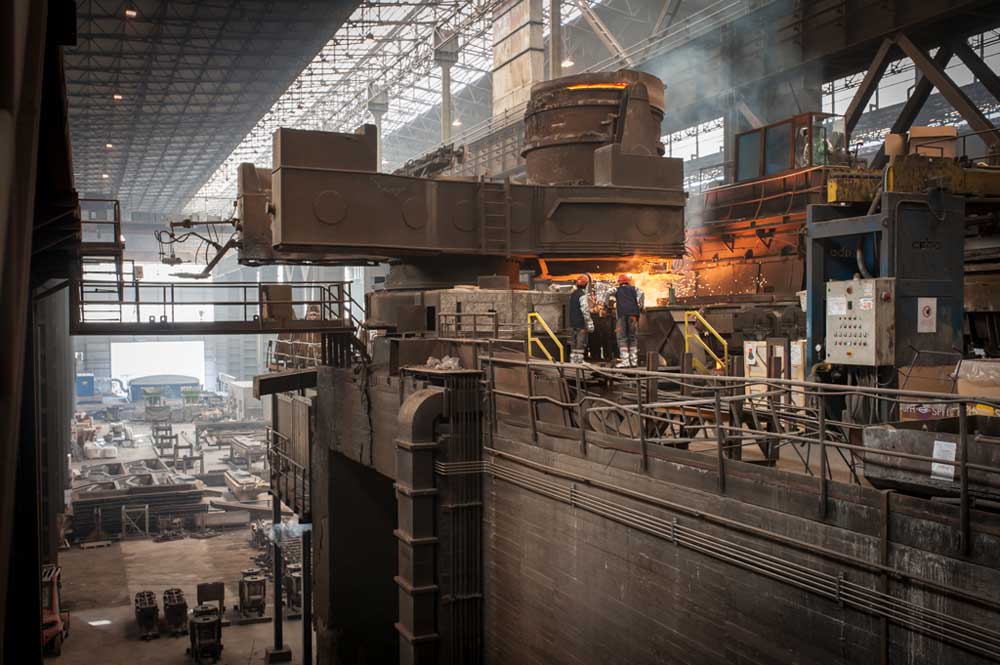
Natural Gas Combustion Optimization
Reducing carbon and NOx emissions in local heating plants.
Ongoing, seeking local partner
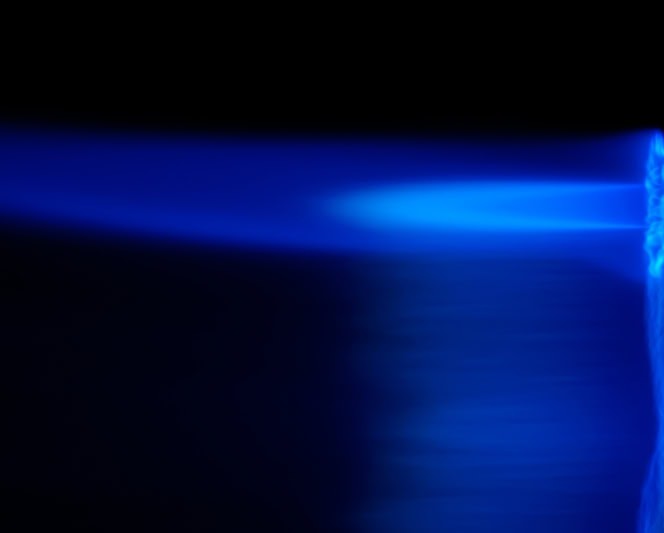
Welding Error Detection
Kontrolinspect, Serbia
ML-based classification of weld defects using historical XRD images.
Ongoing
.jpg)
Alloy Development (Ti Alloys)
Designing new biocompatible/aerospace titanium alloys by combining first-principles calculations, ML, and experimental data.
Ongoing, seeking partners
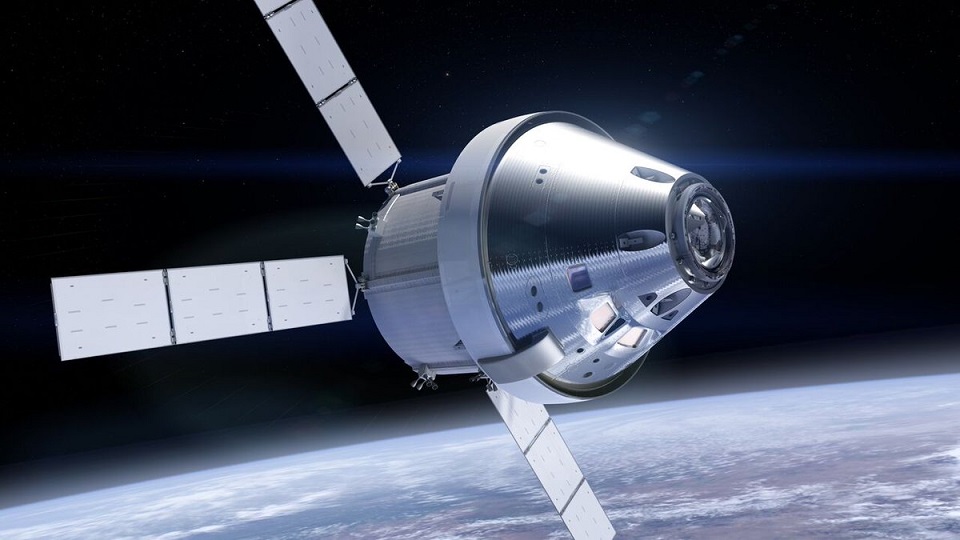
Methods & Technologies
Data-Driven Machine Learning Approach
Outline your step-by-step method (data collection, cleaning, model building, real-time monitoring).
Real-Time Process Monitoring
Short paragraph describing how you integrate sensors, data acquisition, and model updates.
Expert Knowledge
o Emphasize the importance of domain expertise (metallurgy, process engineering) combined with data science.
Contact Us for a Consultation
References & Partners
Selected Publications
V. Manojlović et al. “Machine learning analysis of electric arc furnace process...,” Applied Energy, 307 (2022).
Industry Partners
Metalfer, HBIS, Kontrolinspect, University of Belgrade (Faculty of Technology and Metallurgy)